Aleksandar Anžel is one of the newest members of the MOSLA team. His research focuses on machine learning and data visualization. Both working on analysis and visualizations (Project Area A2), he and Chisom Ezekanna are part of the junior research group instructed by Georges Hattab.
Make sure to read their self-introductions on our team page.
Aleksandar, we’re happy to welcome you to the team! Would you introduce yourself and tell us how you ended up at MOSLA and what’s most interesting for you?
After my Master’s studies, I was hungry for cutting-edge bioinformatics. I felt I had a certain skill-set that is not very common in the bioinformatics community, and I wanted to use those skills and expand them by doing research. German educational system appealed to me, but after finding out about MOSLA, I knew that’s the place where I want to be and continue my studies. The project itself is the most interesting part to me, but the people and the atmosphere in the group are the things that make every task seem easy.
You approach molecular storage with machine & deep learning. What is the thought behind it?
Using DNA as a storage medium is not some new idea. However, the technological progress of certain machine learning methods now allows us to look at DNA as a storage medium from a different angle and possibly to enable us to overcome certain obstacles that arise when using that kind of molecule for such purposes.
You are at home in computer science. Is visualization something you are interested in for a while now? And how do you create your visualisations?
I am fairly new to the visualization community, and I only scraped the tip of the iceberg. For me, the go-to visualization theory book is Visualization Analysis and Design by Tamara Munzner. It has clear guidelines on how to create good visualizations. As for the tool, I am currently creating my visualizations in Python with Altair library, but I am also learning Plotly at the moment.
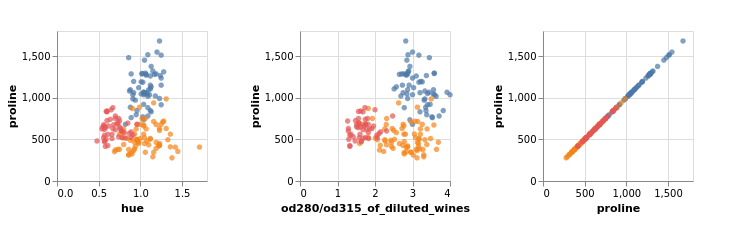
As pointed out, your work at MOSLA not only deals with machine learning but data visualisation as well. What is the advantage of combining machine learning and visualization – and who is the target group of your visualizations for explainable machine learning?
Machine learning quickly becomes too complex even for experts to understand. That is especially true if we are working with deep learning, where neural networks can become huge in a matter of minutes. Good, well-made visualizations can help us grasp abstract and complex things in a way that we can use that knowledge to fix or further improve our methodology and create new hypotheses.
The target groups are all those scientists, students, and hobbyists whose main interest is not machine learning (ML). The idea is to allow a layperson to understand the basics of internal structures of ML models in a visually appealing way. The more people we get into the ML community, the more progress we make in that domain.
Thank you for this close look on your work, Aleksandar!
Now, as a final question: You mention real-world DNA storage applications in your self-introduction. What do you imagine they might look like in the future?
I believe that using DNA as a storage medium is closer than we think. Technology is getting better every day, and what was unimaginable 40 years ago is now our reality. Take, for example, sequencing machines. They were big, bulky, and very expensive. Now we have devices like minION that our colleagues from Oxford created and that can fit in the palm of your hand. For me, that is just amazing. The future is here 🙂
Learn more about Aleksandar Anžel on his Website.